Artificial Intelligence
The Hunyuan-Large Model and MoE Revolution: Transforming AI Intelligence and Efficiency in 2025
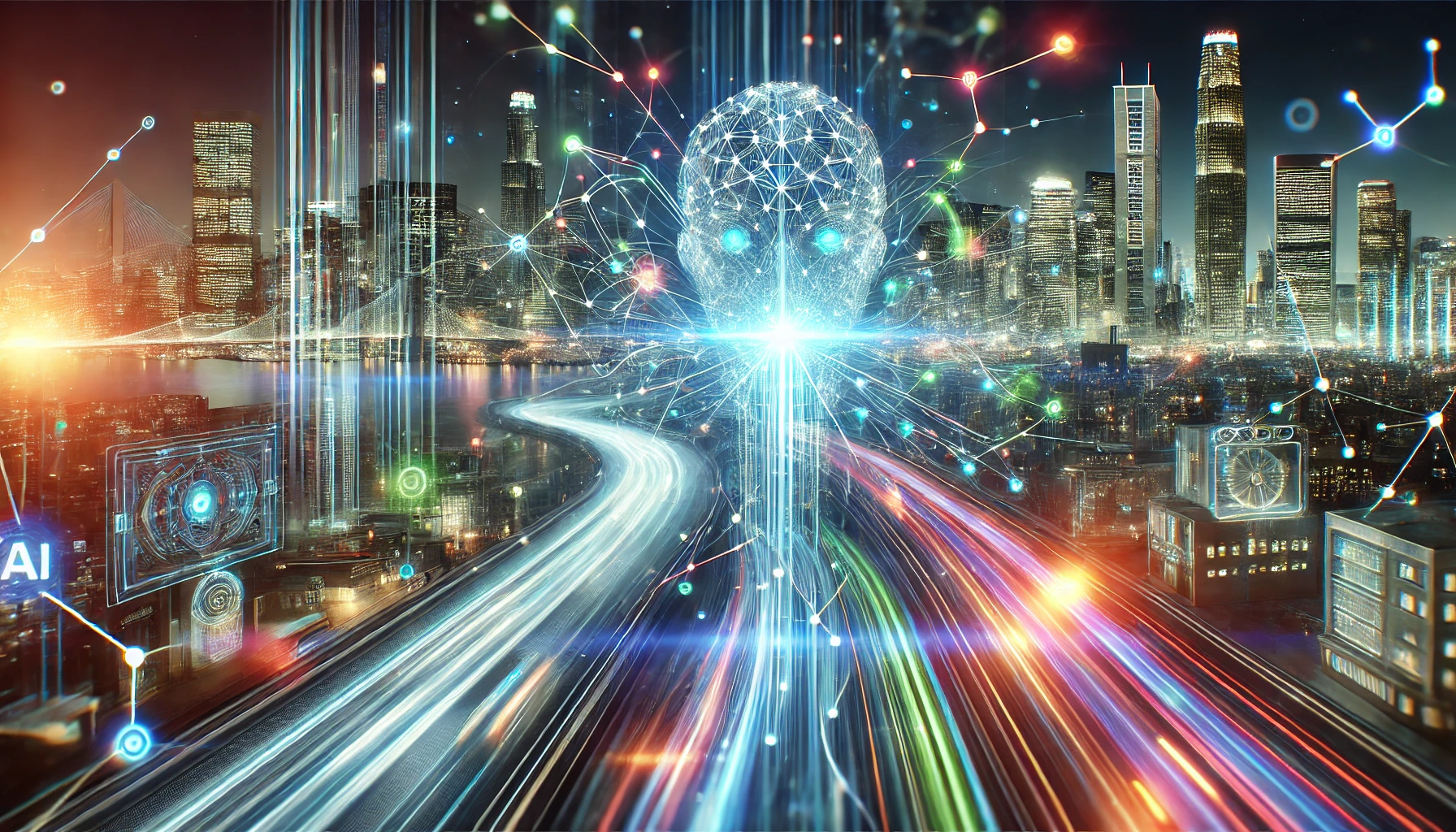
Artificial Intelligence (AI) is evolving at an unprecedented rate. What seemed like a distant vision just a few years ago is now an integral part of our everyday lives. Yet, what we’re seeing now is just the beginning. The real transformation is unfolding behind the scenes, driven by the development of massive AI models capable of performing tasks once thought to be exclusively human. One of the most groundbreaking advancements is Hunyuan-Large, Tencent's cutting-edge open-source AI model.
Hunyuan-Large is among the most advanced AI models ever created, boasting an impressive 389 billion parameters. However, its true innovation lies in the use of the Mixture of Experts (MoE) architecture. Unlike traditional models, MoE activates only the most relevant experts for each task, optimizing efficiency and scalability. This strategy not only enhances performance but also redefines how AI models are designed and deployed, enabling faster and more effective systems.
The Power of Hunyuan-Large
Hunyuan-Large represents a major leap forward in AI technology. Built on the Transformer architecture, which has already proven successful in a range of Natural Language Processing (NLP) tasks, it stands out for its integration of the MoE model. This innovative approach reduces the computational load by activating only the most relevant experts for each task, enabling the model to tackle complex challenges while minimizing resource consumption.
With 389 billion parameters, Hunyuan-Large is one of the largest AI models available today, surpassing earlier models like GPT-3, which has 175 billion parameters. The scale of Hunyuan-Large allows it to handle more advanced tasks, such as deep reasoning, code generation, and long-context processing. It excels at multi-step problems and identifying intricate relationships within large datasets, delivering highly accurate results in even the most demanding scenarios. For example, Hunyuan-Large can generate precise code from natural language prompts, a task that was challenging for previous models.
What sets Hunyuan-Large apart from other AI models is its ability to manage computational resources efficiently. Innovations like KV Cache Compression and Expert-Specific Learning Rate Scaling help optimize memory usage and processing power. KV Cache Compression accelerates data retrieval from the model's memory, enhancing processing times. Expert-Specific Learning Rate Scaling ensures that each part of the model learns at the optimal rate, maintaining high performance across a broad range of tasks.
These advancements give Hunyuan-Large a competitive edge over models like GPT-4 and Llama, especially in tasks requiring deep contextual understanding and reasoning. While models such as GPT-4 excel at natural language generation, Hunyuan-Large’s blend of scalability, efficiency, and specialized processing capabilities allows it to tackle more complex challenges. Its capacity to handle detailed information makes it a valuable tool across diverse applications.
Boosting AI Efficiency with MoE
In AI, more parameters typically translate to greater power, but this approach comes with a downside: higher costs and slower processing times. As AI models grow in complexity, the demand for computational resources skyrockets, leading to increased expenses and delayed processing speeds. This has created a pressing need for a more efficient solution.
Enter the Mixture of Experts (MoE) architecture. MoE revolutionizes the way AI models operate by providing a more efficient and scalable approach. Unlike traditional models, which activate all parts of the model at once, MoE selectively activates only a subset of specialized experts based on the input data. A gating network determines which experts are needed for each specific task, thereby reducing the computational burden without sacrificing performance.
The key benefits of MoE are its improved efficiency and scalability. By activating only the necessary experts, MoE models can process large datasets without significantly increasing resource requirements for every task. This leads to faster processing, lower energy consumption, and reduced costs. In industries like healthcare and finance, where large-scale data analysis is crucial but expensive, MoE's efficiency offers a significant advantage.
Additionally, MoE models can scale more effectively as AI systems become more complex. The number of experts can expand without a proportional increase in computational resources, allowing the model to handle larger datasets and more sophisticated tasks while keeping resource usage in check. As AI continues to integrate into real-time applications, such as autonomous vehicles and IoT devices—where speed and low latency are essential—MoE’s efficiency will become even more valuable.
Hunyuan-Large and the Future of MoE Models
Hunyuan-Large is setting a new benchmark in AI performance. Its ability to handle complex tasks, such as multi-step reasoning and long-context data analysis, outpaces previous models like GPT-4, delivering faster, more accurate results. This makes it particularly well-suited for applications that demand quick, precise, and contextually aware responses.
The potential applications of Hunyuan-Large are vast. In healthcare, it is proving to be a valuable tool for data analysis and AI-driven diagnostics. In natural language processing (NLP), it excels in tasks like sentiment analysis and text summarization, while in computer vision, it is applied to image recognition and object detection. Its strength in managing large datasets and understanding intricate contexts makes it a versatile tool across diverse industries.
Looking ahead, MoE models like Hunyuan-Large will play a pivotal role in the future of AI. As AI models become more sophisticated, the demand for scalable and efficient architectures increases. MoE’s ability to process large datasets without consuming excessive computational resources makes it a more efficient alternative to traditional models. This is particularly important as cloud-based AI services proliferate, enabling organizations to scale operations without the cost and resource demands of older, resource-heavy models.
Emerging trends like edge AI and personalized AI are further expanding the potential of MoE models. In edge AI, data is processed locally on devices instead of being sent to centralized cloud systems, reducing latency and transmission costs. MoE models are ideal for this, enabling real-time, efficient processing on devices. Additionally, personalized AI, powered by MoE, can provide more tailored user experiences, from virtual assistants to recommendation systems.
However, as MoE models grow in power, there are challenges to address. Their size and complexity still demand substantial computational resources, raising concerns about energy consumption and environmental impact. Additionally, ensuring these models are fair, transparent, and accountable is critical as AI continues to advance. Addressing these ethical issues will be essential to maximize the positive impact of AI on society.
The Bottom Line
AI is evolving at a rapid pace, with innovations like Hunyuan-Large and the MoE architecture at the forefront. By improving efficiency and scalability, MoE models make AI more powerful, accessible, and sustainable.
As AI finds applications in fields like healthcare and autonomous vehicles, the demand for smarter, more efficient systems will only grow. With this progress comes the responsibility to ensure AI develops ethically—serving humanity in a fair, transparent, and responsible manner. Hunyuan-Large is a prime example of the future of AI—powerful, flexible, and poised to drive transformative change across industries.